June 2023: Data Implications for AI
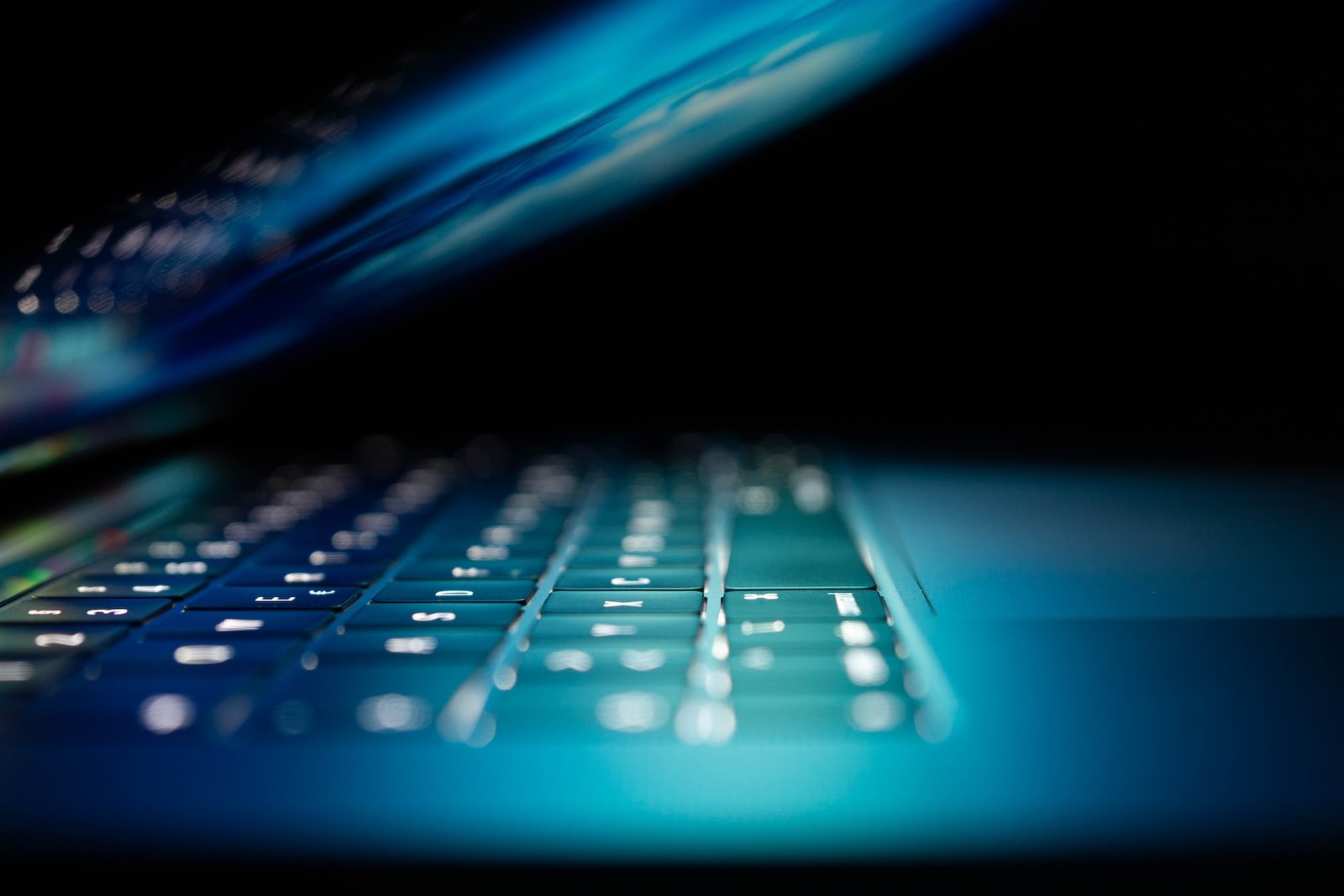
We'll cover new ways for IT managers to be more productive and efficient in managing enterprise data and storage and making the best decisions, to dealing with ever-changing compliance issues, working with departments on data strategies, and understanding the new requirements for data management and AI. On that note, we’re launching our newsletter with a look at the latest wave of AI technologies: what should you and your organization’s leaders consider before putting them into action? Generative AI and its impact on society is an explosive topic right now across conference room tables and mainstream media. Komprise cofounder and CEO Kumar Goswami recently noted: “Enterprises need to be ready for this wave of change and it starts by getting unstructured data prepped, as this data is the critical ingredient for AI/ML.”Segmenting and making data available for these new technologies is just half the battle. Data security, privacy, ownership, lineage, and governance are thorny issues that have come to a head in the last few months. What regulations and policies will enterprise IT leaders need to protect sensitive and proprietary data? Krishna Subramanian, COO and Cofounder of Komprise Clear standards have not yet emerged for data management with AI. Krishna Subramanian, co-founder, and COO, writes in Datanami on the topic, exploring why enterprises should tread carefully and ensure they clearly understand the data exposure, data leakage, and potential data security risks before using AI applications. Here’s an excerpt: Recently, many in the tech and security community have sent out warning bells due to a lack of understanding and sufficient regulatory guardrails around the use of AI technology. We are already seeing concerns about the reliability of outputs from the AI tools, IP and sensitive data leaks, and privacy and security violations. Samsung’s incident with ChatGPT made headlines after the tech giant unwittingly leaked its own secrets into the AI service. Samsung is not alone: A study by Cyberhaven found that 4% of employees have put sensitive corporate data into the large language model. Many are unaware that when they train a model with their corporate data, the AI company may be able to reuse that data elsewhere. And as if we didn’t need more fodder for cyber criminals, there’s this revelation from Recorded Future, a cybersecurity intelligence firm: “Within days of the ChatGPT launch, we identified many threat actors on the dark web and special-access forums sharing buggy but functional malware, social engineering tutorials, money-making schemes, and more — all enabled by the use of ChatGPT.” On the privacy front, when an individual signs up with a tool like ChatGPT, it can access the IP address, browser settings, and browsing activity—just like today’s search engines. But the risk is higher, because “without an individual’s consent, it could disclose political beliefs or sexual orientation and could mean embarrassing or even career-ruining information is released,” according to Jose Blaya, the Director of Engineering at Private Internet Access. The article goes into detail on three areas of focus to ensure proper data governance with AI programs: Data governance and transparency with training data Data segregation and data domains The derivate works of AI Komprise CEO Kumar Goswami covers a few key practices to get started on your unstructured data management infrastructure journey in the AI era, in this article on The Cloud Awards site: There is much to yet understand about the potential for AI and its impact on not only work and economic output but our personal lives. Enterprises need to be ready for this wave of change – and it starts by first getting a complete picture of the unstructured data often locked in storage silos and disconnected file systems across the enterprise. New data management technologies and strategies will enable the creation of automated ways to index, segment, curate, tag and move unstructured data continuously to feed AI and ML tools. Unforeseen changes to society, fueled by AI, are coming soon and you don’t want to be caught flat-footed. Is your organization ready? To take advantage of the AI/ML innovation landscape, here are a few key practices to start you on your unstructured data management infrastructure journey: 1. Get full visibility so you can optimize and leverage your data. Organizations often don’t have the full picture of their unstructured data, which leads to the fact that most data behind the firewall is not used much less leveraged for competitive gain. IT leaders and other data stakeholders often don’t know which data is the most valuable in terms of access frequency or ownership, or where there are hidden silos of unused data eating up expensive storage. Organizations typically actively use only 20 percent of the data they have in storage. Therefore, IT could move a large percentage of data to cheaper storage based on usage. Of course, deleting data altogether is sometimes appropriate. With an analytics approach to data management, IT leaders can develop a nuanced strategy that considers current and future data value. The first step is to recognize your current situation and find ways to move from a storage-centric to a data-centric approach. 2. If you aren’t indexing your data today, that’s a problem. A seminal barrier to data analytics is finding the precise data you need to mine. Most people in “data” jobs — data analysts, data scientists, researchers, marketers — spend most of their time looking for the data that will fit a project’s requirements. One of our customers told us how their researchers from one location used to call those in another to find the data they need for experiments. This doesn’t scale. Data indexing is a powerful way to categorize all your unstructured data across your enterprise and make it searchable by key metadata such as file size, file extension, date of file creation, date of last access, and custom (user-created) metadata such as project name or keyword (such as an experiment name or instrument ID). Creating a global data index gives central IT and departmental IT teams and data researchers the equivalent of Google Search across your enterprise. This way, you don’t have to physically move your data; silos aren’t the issue if you can look across them from your data center to the cloud to find and use what you need. 3. Make new uses of data while still being cost-efficient. Now that your data is indexed, users can find precisely the data sets they need and create policies to automate the movement of data in a query to the location of choice—such as a cloud data lake for AI analysis. This requires automation and a simple way to connect the dots so you can deliver the right data to the right place (and to the right people or applications) for action. Imagine creating custom workflows that enrich and optimize your data! For example: what if you could tag and automatically tier instrument data to low-cost cloud storage as it is created? Cloud AI and ML tools can then ingest the data for analysis. Once the analysis is complete, an unstructured data management solution can automatically move the data to a colder, cheaper tier. Meanwhile, all of this happens automatically and at significantly lower costs to IT. James Maguire, editor-in-chief of eWeek interviews Komprise COO Krishna Subramanian about the pressing data security problems with AI, the future of managing data with AI and how Komprise plays a role. Here are some key exchanges from their conversation, which you can listen to here. Says Krishna: “Things like ChatGPT have captured our imagination because they seem human. But they are generating new content using very good pattern matching based on learning models that are trained. Generative IT sounds extremely intelligent and creative because language follows certain patterns. Yet there are lot of data management issues because it's running on data. We do have to understand its boundaries especially concerning data ownership, privacy security, and leakage. Companies built on proprietary IT can find themselves being sued on stuff that they thoughts they’d never be liable for or sharing leakage of proprietary IT.” Maguire: Can Komprise help? Krishna: “All of the data that AI uses is unstructured data. It’s not data in databases but data from the internet, videos, and documents. Analyzing what data is being used by whom, how is your data being shared, what access controls you have, and moving large amounts of data in and out of a large learning model. These are the kind of things that Komprise does and we are still learning.” Maguire: How do you see AI evolving? “Standards are definitely needed. The EU is already creating some and the U.S. is starting on this. There is still a lot to do concerning the data. Data management must be front and center. I think there will be a tighter understanding of this and there will be a regulatory framework for these solutions to operate. Government has to create this framework. In an industry that has the potential for great good and great harm, you need regulation. There will likely be some defacto standards that businesses will start adhering to on their own and regulation will follow.”Data management implications for Generative AI
The AI/ML Revolution: Data Management Needs to Evolve
eWeek Podcast: Data Management and AI